A data lakehouse is a hybrid data architecture that combines the benefits of two traditional data management systems: data warehouses and data lakes. First, we’ll examine what each of those systems is and why they both have their pros and cons for data operations.
Data warehouse
Data warehouses were the earliest data management systems built to accommodate specific, structured data. They gave data scientists a central repository to easily locate and analyze specific datasets. Modern data warehouses fulfill much the same role and hold selected data with a known business use case. Data scientists access and use this reliable, structured data to create dashboards and publish reports.
However, data warehouses can hold data operations back for a few reasons. First, their lack of scale and the time it takes to perform necessary ETL and cleaning processes can slow business insight (BI) and lead to high maintenance costs. Second, they create significant business risks through the creation of extra copies of data and vendor lock-in.
Data lake
A data lake is an architecture developed to handle the considerable expansion of the scope of data collection. Data lakes allow virtually unlimited storage for all data types and are essential for the modern mass collection of unstructured or semi-structured data.
One drawback is that there is lower quality control on the data. Data ends up stored because it “might” have a business use in the future. Relevant data becomes more difficult to identify and gather for analysis. Ultimately, data lakes are a cheaper, faster storage option that can be paired with AI to provide some general insights.
What does a data lakehouse deliver?
As you can see, both major data management systems have their purposes but also their drawbacks for data operations. As a result, many organizations combine multiple data warehouses and a data lake, then perform ETL processes to copy the necessary data assets for analysis and use. However, this still doesn’t solve the problem of DataOps needing a high-speed and flexible system that can support diverse operations. This is where a data lakehouse system comes in.
Leveraging the capabilities of data virtualization, which creates an interoperable layer of virtual data that sits over data storage, data scientists can combine the best features of the data lake and warehouse to create the data lakehouse. A data lakehouse provides the same data management features of data warehouses with the performance, flexibility, and lower costs of data lakes.
This means data scientists can take advantage of a range of critical features in one place, including:
- Tighter data governance: Data governance is an essential element of DataOps and helps reduce overall risk. Since a data lakehouse performs all actions on a virtual data layer, comprehensive data governance protocols can be applied to data where it resides and throughout its processing. Giving data administrators greater controls helps them ensure compliance and reduces the organizational risk created by data usage.
- Secure data sharing: Collaboration with other organizations or working with third-party analytics firms previously involved considerable risk of data breaches, as sharing data meant losing control over what happened to it. Data lakehouses allow data owners to retain complete custody over their data assets and what happens to them. A useful analogy is that of using Netflix or Spotify—you can have full access to their content, but can’t ever keep it or claim ownership of it to use for purposes outside your contract.
- Better security: Creating multiple copies of data and siloing data assets creates risk for data admins who can’t be sure of the location and security of all data. Data lakehouses remove this problem as data never has to be moved from its storage location or copied. Additionally, all processing is performed in containerized secure execution environments, further reducing the attack surface of data operations.
- Agile data operations: An effective agile DataOps function requires insight velocity and data I/O flows cleaned of friction points. Such data streaming is possible through data lakehouses as unstructured or semi-structured data can be delivered straight to analysis with machine learning tools. This enables real-time reporting and dashboards that serve real-time applications. Removing the need for ETL processes also greatly reduces your data function’s time-to-insight.
- Better ROI: Data warehouses are expensive in terms of storage and resources required to maintain and perform operations such as cleaning, processing, and migrations. A major advantage of data lakehouses is that they cut these costs without losing the functionality of your data function, all while improving on insight velocity which prevents value decay on your data.
Data lakehouses: The best of both worlds
In the past, data functions have had to deploy dispersed data management architecture, often featuring multiple, siloed, data warehouses and a data lake for their larger, unstructured data. This meant that actual analysis was slow and had to be proceeded by lengthy ETL and cleaning processes, while data lakes lacked the functionality to allow greater analysis to be performed on incoming data.
Modern data lakehouses give data functions the best of both worlds: the speed, size, and flexibility of data lakes along with the analysis capacity and governance controls of data warehouses. Data lakehouses are enabled by virtual data platforms, which create a secure and interoperable data layer over all your stored data, allowing DataOps to easily locate and perform analysis on the data they want wherever it resides, without needing to copy it or perform ETL.
To find out how Intertrust Platform creates data lakehouses that improve data operations, ensure regulatory compliance, and deliver better ROI, you can read more here or talk to our team.
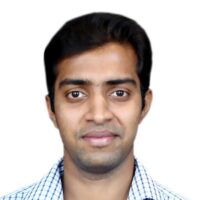
About Abhishek Prabhakar
Abhishek Prabhakar is a Senior Manager ( Marketing Strategy and Product Planning ) at Intertrust Technologies Corporation, and is primarily involved in the global product marketing and planning function for The Intertrust Platform. He has extensive experience in the field of new age enterprise transformation technologies and is actively involved in market research and strategic partnerships in the field.